Measurement scale (NOIR)
- IJNGP
- Mar 29, 2023
- 9 min read
Introduction to Statistics
We all deal with statistics on a daily basis. One only needs to look at their bank account or the newspaper's baseball box scores to get evidence of statistics. Statistics by themselves don't elicit fear. For instance, most people won't feel anxious when they see a player's batting average on the television. The "batting average" is a number, but since we understand what it means and how to interpret it, we don't find it to be very alarming. Simply since statistics is a tool we are unfamiliar with, the thought of them frequently causes uneasiness. So let's analyse what the term statistic actually means. A formal definition is given by Kuzma, a set of methods and strategies for gathering, organising, analysing, interpreting, and presenting information that may be expressed quantitatively.
Descriptive statistics
They are used to organise or summarise a particular set of measurements. Alternatively said, a descriptive statistic will provide a description of that collection of measures. An example of a descriptive statistic is the player's batting average in baseball. The ability to hit a baseball at any period in the past is described.
Inferential statistics
It uses data gathered from a sample to make inferences about the larger population from which the sample was drawn. For instance, during an election, a small sample of individuals is surveyed, and the results are subsequently used to characterise the voting public as a whole.
Introduction to Measurement
Typically, when someone hears the word measurement, they could picture measuring a quantity of something (ie. a cup of curd) or the length of something (such as the length of a piece of cloth). This is an insufficient usage of the word measurement.
The term "measurement" is used more loosely and is better referred to as "scales of measurement" in statistics. Scales of measurement are methods for defining and classifying variables and numbers. The four scales of measurement created by psychologist Stanley Stevens are — nominal, ordinal, interval, and ratio. Each scale of measurement has certain characteristics, which in turn dictate whether or not specific statistical analyses may be used. The qualities that are assessed include identity, magnitude, equal intervals, and a minimum value of zero.
Properties of Measurement
Identity: Identity implies each value having a unique meaning.
Magnitude: Magnitude means that the values have an ordered relationship to one another, so there is a specific order to the variables.
Equal intervals: Equal intervals represent that data points along the scale are equal, so the difference between data points one and two will be the same as between data points five and six.
A minimum value of zero: A minimum value of zero expresses that the scale has a true zero point. Degrees, for example, can fall below zero and still have meaning. But if you weigh nothing, you don’t exist.
Four Scales of Measurement
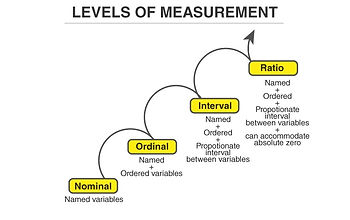
1. Nominal Scale
Nominal scale is the first level of measurement. Since this scale is a rudimentary form, many people would readily argue that it is ludicrous to give the process of assigning numerals the dignity suggested by the term measurement. This point cannot possibly be contested because giving things names is a completely random process.
The nominal scale of measurement determines the identity property of data. Although this scale has certain features, there is no numerical value associated with it. For purposes of categorising or identifying things, the numbers serve as "tags" or "labels." Typically, a nominal scale is used to deal with non-numeric variables or numbers that have no inherent significance. Although the data may be broken up into categories, it cannot be multiplied, divided, added to, or subtracted from. A measurement of the variation between data points is likewise
For instance, there are two classes for the variable "gender": males and females. Gender is a nominal variable since neither it nor these classes have any quantitative properties. Additionally, eye colour and place of birth are instances of nominal data.
There are 3 categories of nominal scale -
Nominal with order: Some nominal data can be sub-categorized in order, such as “cold, warm, hot, and very hot.”
Nominal without order: Nominal data can also be sub-categorised as nominal without order, such as male and female.
Dichotomous: Dichotomous data is denoted by having only two categories or levels, such as “yes’ and ‘no’.
Characteristics of Nominal Scale
A nominal scale variable is divided into two or more categories. The response should fit into one of the classifications in this measuring procedure.
It is qualitative, they do not have quantitative properties. The numbers are used here to identify the objects only since no comparison can be made as to which one is higher than the other.
The numbers don’t define the object characteristics. The only permissible aspect of numbers in the nominal scale is “counting.”
2. Ordinal Scale
The ordinal scale is the second level of measurement. Ordinal symbols ‘order’ meaning data is ordered and ranked without determining how different they are from one another. Ordinal data are sometimes referred to as qualitative or categorical data. It can be graded, classified, and given names. Although each value is graded, there is no information indicating what sets the categories apart from one another. These values can’t be added to or subtracted from. Here, the relationships are only understood in terms of levels like greater or lower, more or less rather than actual numbers.
Ordinal scales make up the majority of the commonly and successfully used scales by psychologists. Since they show rank ordering, Likert type scales are another form of ordinal data. This may be seen in satisfaction data points after a race, where "1=happy, 2=neutral, and 3=unhappy" are the possible outcomes. First place, second place, or third place indicate the runners' finishing positions, but they do not indicate how much in front of second place the first-place finisher was.
Characteristics of the Ordinal Scale
This scale displays the relative ranking of the variables along with the information provided by the nominal scale.
There are distinct classes but these classes have a natural ordering or ranking. The difference between these classes is on the basis of magnitude.
Ordinal scales do not assume that the intervals are equal.
3. Interval Scale
The third level of measurement is the interval scale. Although the characteristics of nominal and ordered data are present in this scale, there is quantifiable variation between the data points. The variables' actual positions in the order as well as their differences are both displayed by this form of data. The interval scale's data points have the same difference between them. For instance, the difference between 10 and 20 degrees and 20 and 30 degrees is the same. Unlike the previous two scales, which are solely intended to express qualitative values, this scale is used to quantify the differences between variables. It is crucial to remember that while the values can be multiplied or divided, they cannot be added to or subtracted from one another.
Except for those that assume knowledge of a "real" zero point, almost all common statistical measures are usable here. The fact that this scale is unaffected by the addition of a constant demonstrates that the zero point on an interval scale is a matter of convenience or convention. Zero on the ordinal scale indicates that there is no data. Zero on an interval scale has significance; for instance, if you measure in degrees, zero has a temperature.
An interval variable is, for instance, the temperature in Celsius. Meaningful comparisons include stating that 25 degrees Celsius is three degrees hotter than 22 degrees Celsius and that 17 degrees Celsius is three degrees hotter than 14 degrees Celsius. However, take note that 0 degrees Celsius does not have significance in nature. That is, 0 degrees Celsius does not indicate that there is no heat present!
Characteristics of Interval Scale:
This scale is quantitative in nature as it can quantify the difference between the values.
The mean and median of the variables can be calculated.
You can subtract the values between the variables to understand the difference between them.
This scale designates an equal interval ordering.
4. Ratio Scale
The ratio scale is the 4th level of measurement scale, which is quantitative. It is a type of variable measurement scale. It allows researchers to compare the differences or intervals. The ratio scale has a unique feature. It possesses the character of the origin or zero points. Ratio scales of measurement include properties from all four scales of measurement. The data is nominal and defined by an identity, can be classified in order, contains intervals and can be broken down into exact values. Weight, height and distance are all examples of ratio variables. Data in the ratio scale can be added, subtracted, divided and multiplied.
Even though they are uncommon, psychological magnitude ratio scales are not completely unheard of. The British committee's discussion of the Sone scale is one such system that was specifically designed to allow human observers to assess the loudness ratios of pairs of tones. This kind of scale is most frequently seen in physics, and it can only be determined using operations for all four relations—equality, rank-order, equality of intervals, and equality of ratios—when those operations are available. Once such a scale has been built, the only way to change its numerical values (such as from inches to feet) is by multiplying each value by a constant. The zero value on certain scales may never be achieved, but an absolute zero is always assumed.
The interval scale and the ratio scale both represent quantity and also have equivalent units, making them comparable scales for measurements. They do, however, vary since the ratio scale also contains an absolute zero. The data has no value point if the value is zero. An example of this is height or weight, as one cannot be 0 cm tall or 0 kgs, or be negative centimetres or negative kgs. Calculating shares or sales are examples of how to utilise this scale. Data scientists can make the greatest use of ratio data points out of all the data kinds on the scales of measurement.
Characteristics of Ratio Scale:
Ratio scale has a characteristic of absolute zero.
The values cannot be determined in negative because of its zero-point feature.
It affords unique opportunities for statistical analysis. The variables can be orderly added, subtracted, multiplied, and divided.
Mean, median, and mode can be calculated using the ratio scale.
Ratio scale has unique and useful properties. One such feature is that it allows unit conversions like kilogram to calories, gram to calories, etc.
Table 01 - Only ratio scale meets all four criteria for properties of levels of measurement
Scale | Indicates Difference | Indicates Direction of Difference | Indicates Amount of Difference | Absolute Zero |
Nominal | ✓ | | | |
Ordinal | ✓ | ✓ | | |
Interval | ✓ | ✓ | ✓ | |
Ratio | ✓ | ✓ | ✓ | ✓ |
Table 02 - Other properties of the four levels of measurement
Scale | Basic Expirical Operations | Mathematical Group Structure | Permissible Statistics |
Nominal | Determination of equality | Permutation group x’=f(x) where f(x) means any one-to-one substitution | Frequency Chi-square Mode Contingency correlation |
Ordinal | Determination of greater or less | Isotonic group x’=f(x) where f(x) means any monotonic increasing function | Median Percentiles Rank-order correlation |
Interval | Determination of equality of intervals or differences | General linear group x’=ax+b | Mean Standard deviation Rank-order correlation Product-moment correlation |
Ratio | Determination of equality of ratios | Similarity group x’=ax | Coefficient of variation |
Conclusion
In statistical terms, data is individual pieces of factual information recorded, and it is used for the purpose of the analysis process. Data can be divided into 2 types, namely, qualitative and quantitative.
Qualitative data
It is non-numerical. It refers to information about qualities, or information that cannot be measured. It’s usually descriptive and textual. Examples include someone’s hair colour. Nominal and ordinal scales fall under this category.
Quantitative data
It includes numbers. It’s used to define the information that is quantifiable or can be counted. Quantitative data can be further broken down into 2 categories, discrete and continuous.
Discrete data is a whole number that can’t be further broken down into individual parts or fractions. For instance, the number of family members – one can have three members but not two-and-a-half members.
Continuous data describes values that can be further broken down into different parts, fractions, and decimals. This data can be measured easily. For example, height, weight, and time can also be broken down into half a second or half an hour. Interval and ratio scales fall under this subcategory.

There are 2 branches of statistics that can help us further understand the levels of measurement in a more clear and defined manner.
Parametric data
Signifies that the data meets certain requirements with respect to the parameters of the population. Additionally, the numbers can be added, subtracted, multiplied, and divided. Parametric data are analysed using statistical techniques identified as Parametric Statistics. As a rule, there are more statistical technique options for the analysis of parametric data. Since they may be stated quantitatively and can specify the intervals between values, interval and ratio scales are considered parametric.
Nonparametric data
It means that the data does not fit into the requirements with respect to the parameters of the population. Since they lack those parameters, they can not be added, subtracted, multiplied, or divided. Nonparametric data are analysed by using Nonparametric Statistics. Due to the lack of a wide range of statistical technique alternatives for analysis, nonparametric statistics are viewed as less powerful than parametric statistics. Nominal and ordinal scales are categorised as nonparametric as they are variables without quantitative values, as we can recollect from what we have learned about the characteristics of the various scales.
References
Mangal, S. K. (2004). Statistics in Psychology and Education. Paperback.
(2021, March 22). Scales of measurement. BYJUS. https://byjus.com/maths/scales-of-measurement/
(2020, January 30). Types of data & the scales of measurement. https://studyonline.unsw.edu.au/blog/types-of-data
Stevens, S. S. (1946). On the Theory of Scales of Measurement. Science, 103(2684), 677–680. doi:10.1126/science.103.2684.677
The Article How to write a Research Proposal has been contributed by Diksha Jain and Peer Reviewed by Sanika Sharma. Diksha Jain is a proactive and self-motivated individual with a very keen interest in the field of psychology. Owing to the importance of mental health in today's times has helped her gain a vision of helping people thrive in their lives in its truest sense.
Sanika Sharma is a Psychology Graduate. She is an avid reader and a curious learner. She is inclined towards a holistic understanding of concepts and thus inclines towards interdisciplinary research.
They are a part of the Global Internship Research Program (GIRP). GIRP is an IJNGP initiative to encourage young adults across our globe to showcase their research skills in psychology and to present them in creative content expression.